Analyzing the Biblia Hebraica Stuttgartensia with Text Fabric in Python
— Albert De La FuenteI am very curious about Hebrew, specially the way the language is hierarchically designed. Put very simply, three letter words are commonly known as trilateral roots and the rest of the words are variation of these three letters having a common root word and having related meaning somehow, thus creating a “family” if you will. I am not going to get into these details since it is not the scope of this post.
Long story short, the journey of trying to learn Hebrew started probably between 2006 to 2008 and I haven’t had much success at it. Or lets say that the expectations don’t match reality due to lack of consistent effort on my side.
I thought that I could try to somehow start with the most common words in the scriptures and that is what motivated to parse the Aleppo codex and analyze its content in python. I quickly learned that my approach was rather naive given the prefixes that causes variations to the words, even thought the meaning of the word itself is the same.
This blog post is a second attempt to tackle the same problem but with a more sophisticated and accurate approach using Text Fabric.
A corpus of ancient texts and (linguistic) annotations represents a large body of knowledge.
Text-Fabric is a Python package for processing and access a corpus of ancient text and linguistic annotations. In this specific case I am using the Hebrew Bible Database, containing the text of the Hebrew Bible augmented with linguistic annotations compiled by the Eep Talstra Centre for Bible and Computer from the VU University Amsterdam.
The text is based on the Biblia Hebraica Stuttgartensia edited by Karl Elliger and Wilhelm Rudolph, Fifth Revised Edition, edited by Adrian Schenker, © 1977 and 1997 Deutsche Bibelgesellschaft, Stuttgart.
The text-fabric version has been prepared by Dirk Roorda Data Archiving and Networked Services, with thanks to Martijn Naaijer, Cody Kingham, and Constantijn Sikkel.
It is amazing to see the work these researchers did compiling all this data and making it public for free. I am very thankful for it.
I am using a literate programming approach with Doom Emacs and org-mode. This blog post is a bit more technical oriented. So it is okay if you pass through the code or if you don’t understand some of it.
Create the virtual environment #
This snippet will create a virtual environment and install some libs. Due to some incompatibilities with the word cloud lib, I had to downgrate do Python 3.6.
#virtualenv ~/.workon-home/venv-textfabric
virtualenv --python=/usr/bin/python3.6 ~/.workon-home/venv-textfabric
cd ~/.workon-home/venv-textfabric
source ./bin/activate.fish
pip install text-fabric pandas requests
Activate the virtual environment #
Use C-c
here on Doom Emacs
(pyvenv-activate "~/.workon-home/venv-textfabric")
Load the ETCBC/bhsa dataset #
Use run-python
and ober-eval-block-in-repl
to evaluate each block (C-c r
at the time being). For the sake of a more practical approach to writing using
literate programming the output of the blocks will follow the code. The
documentation of the BHSA dataset can be found here.
from tf.app import use
import os
import collections
from itertools import chain
A = use("ETCBC/bhsa", hoist=globals())
A.indent(reset=True)
A.info("counting objects ...")
for otype in F.otype.all:
i = 0
A.indent(level=1, reset=True)
for n in F.otype.s(otype):
i += 1
A.info("{:>7} {}s".format(i, otype))
A.indent(level=0)
A.info("Done")
<IPython.core.display.HTML object>
<IPython.core.display.HTML object>
<IPython.core.display.HTML object>
<IPython.core.display.HTML object>
This is Text-Fabric 9.5.2
Api reference : https://annotation.github.io/text-fabric/tf/cheatsheet.html
122 features found and 0 ignored
<IPython.core.display.HTML object>
<IPython.core.display.HTML object>
<IPython.core.display.HTML object>
<IPython.core.display.HTML object>
0.00s counting objects ...
| 0.00s 39 books
| 0.00s 929 chapters
| 0.00s 9230 lexs
| 0.00s 23213 verses
| 0.00s 45179 half_verses
| 0.00s 63717 sentences
| 0.00s 64514 sentence_atoms
| 0.00s 88131 clauses
| 0.00s 90704 clause_atoms
| 0.01s 253203 phrases
| 0.01s 267532 phrase_atoms
| 0.01s 113850 subphrases
| 0.02s 426590 words
0.07s Done
Analyzing the type of word structures available #
Text-Fabric allows to query the “types” of words. We can do this by using:
print(F.sp.freqList())
(('subs', 125583), ('verb', 75451), ('prep', 73298), ('conj', 62737), ('nmpr', 35607), ('art', 30387), ('adjv', 10141), ('nega', 6059), ('prps', 5035), ('advb', 4603), ('prde', 2678), ('intj', 1912), ('inrg', 1303), ('prin', 1026))
Defining some help functions #
These functions will facilitate the processing of the data. The first one will
get the occurrences of a specific type of lexeme. I am not a linguist so I am
learning on the go and I will try to explain it easily. The most common lexeme
verb is אמר
(say). It appears 5307 times. We can see that Yah indeed wants to
communicate and instruct us.
A specific instance of that verb has other properties (node features) like morphology which contains the verbal stem (qal, piel, nif, hif), the verbal tense (perf, impf, wayq) and gender (m, f) among other information. A lexeme is the representation of that word (verb in this case) in the broad aspect, i.e regardless of the verbal stem, tense, gender, etc.
import pandas as pd
def get_lexeme_by_type(lex_type):
rows = []
A.indent(reset=True)
for w in F.otype.s("lex"):
if F.sp.v(w) != lex_type:
continue
row = {'w': w,
'freq_lex': F.freq_lex.v(w),
#'sp': F.sp.v(w),
'lex_utf8': F.lex_utf8.v(w),
'gloss': F.gloss.v(w),
#'phono': F.phono.v(w),
#'g_word_utf8': F.g_word_utf8.v(w),
#'g_lex_utf8': F.g_lex_utf8.v(w),
#'g_cons_utf8': F.g_cons_utf8.v(w),
#'gn': F.gn.v(w),
#'nu': F.nu.v(w),
#'ps': F.ps.v(w),
#'st': F.st.v(w),
#'vs': F.vs.v(w),
#'vt': F.vt.v(w),
#'book': F.book.v(w),
}
rows.append(row)
df = pd.DataFrame(rows)
return(df)
def export_df_to_org_table(input_df, rows_qty):
input_df["lex_utf8"] = input_df.apply(lambda x: remove_diacritics(x["lex_utf8"]), axis=1)
output_df = input_df.head(rows_qty)
return([list(output_df)] + [None] + output_df.values.tolist())
Most frequent prepositions #
df_preps = get_lexeme_by_type('prep')
df_cloud = df_preps.sort_values(by=['freq_lex'], ascending=False)
print_df = export_df_to_org_table(df_cloud, 100)
w | freq_lex | lex_utf8 | gloss |
---|---|---|---|
1437629 | 20069 | ל | to |
1437602 | 15542 | ב | in |
1437606 | 10987 | את | <object marker> |
1437639 | 7562 | מן | from |
1437615 | 5766 | על | upon |
1437644 | 5517 | אל | to |
1437693 | 2902 | כ | as |
1437843 | 1263 | עד | unto |
1437805 | 1049 | עם | with |
1437865 | 878 | את | together with |
1443534 | 378 | ל | to |
1443536 | 345 | די | <relative> |
1438236 | 272 | למען | because of |
1445292 | 226 | ב | in |
1438491 | 142 | כמו | like |
1443543 | 119 | מן | from |
1445269 | 104 | על | upon |
1443531 | 63 | כ | like |
1445265 | 35 | עד | until |
1445281 | 22 | עם | with |
1438337 | 17 | בלעדי | without |
1443082 | 9 | במו | in |
1444752 | 4 | למו | to |
1445487 | 1 | ית | <nota accusativi> |
1445919 | 1 | לות | with |
Fair enough, this is a good starting point to learn some words.
Most frequent names (people and places) #
Now lets see the 30 most cited names in scriptures
df_nmpr = get_lexeme_by_type('nmpr')
df_cloud = df_nmpr.sort_values(by=['freq_lex'], ascending=False)
print_df = export_df_to_org_table(df_cloud, 30)
w | freq_lex | lex_utf8 | gloss |
---|---|---|---|
1437714 | 6828 | יהוה | YHWH |
1438941 | 2506 | ישראל | Israel |
1441856 | 1075 | דוד | David |
1438822 | 819 | יהודה | Judah |
1439439 | 766 | משה | Moses |
1438103 | 681 | מצרים | Egypt |
1441150 | 643 | ירושלם | Jerusalem |
1438343 | 438 | אדני | Lord |
1439060 | 406 | שאול | Saul |
1438702 | 349 | יעקב | Jacob |
1439473 | 347 | אהרן | Aaron |
1442014 | 293 | שלמה | Solomon |
1438115 | 262 | בבל | Babel |
1439714 | 218 | יהושע | Joshua |
1438843 | 213 | יוסף | Joseph |
1438262 | 182 | ירדן | Jordan |
1439203 | 180 | אפרים | Ephraim |
1438512 | 180 | מואב | Moab |
1438408 | 175 | אברהם | Abraham |
1439001 | 166 | בנימן | Benjamin |
1442009 | 154 | ציון | Zion |
1437762 | 151 | אשור | Asshur |
1438157 | 149 | ארם | Aram |
1439200 | 146 | מנשה | Manasseh |
1441940 | 145 | יואב | Joab |
1440774 | 140 | שמואל | Samuel |
1438890 | 134 | גלעד | Gilead |
1442693 | 129 | ירמיהו | Jeremiah |
1442394 | 109 | שמרון | Samaria |
1441986 | 109 | אבשלום | Absalom |
And now lets generate a cloud image for in the Hebrew
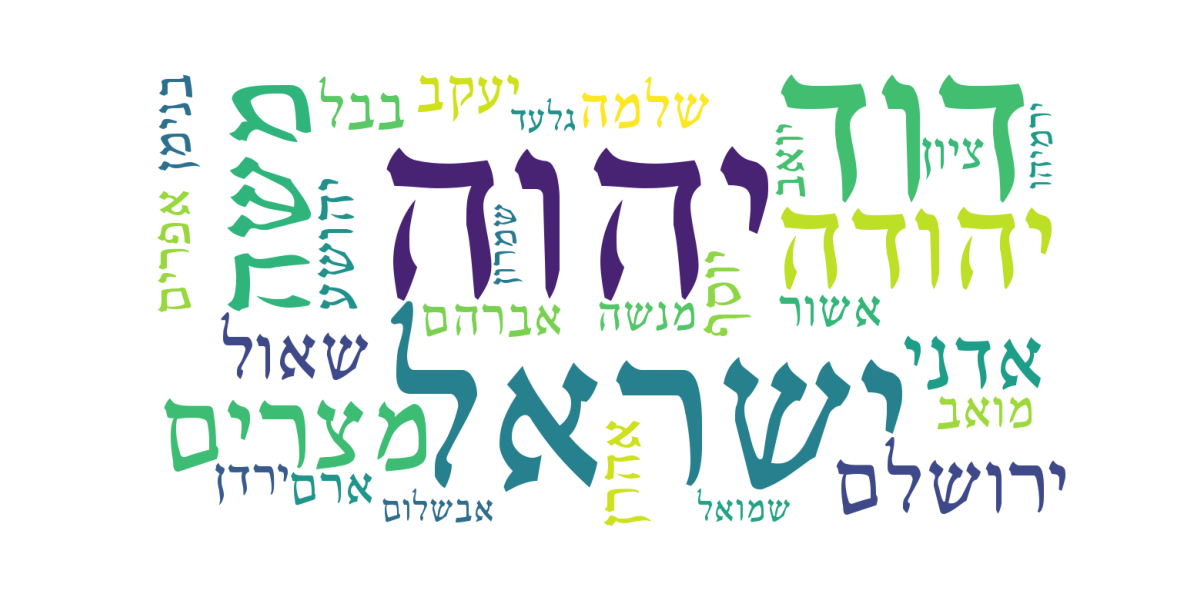
And also a cloud image for the English translations of those names
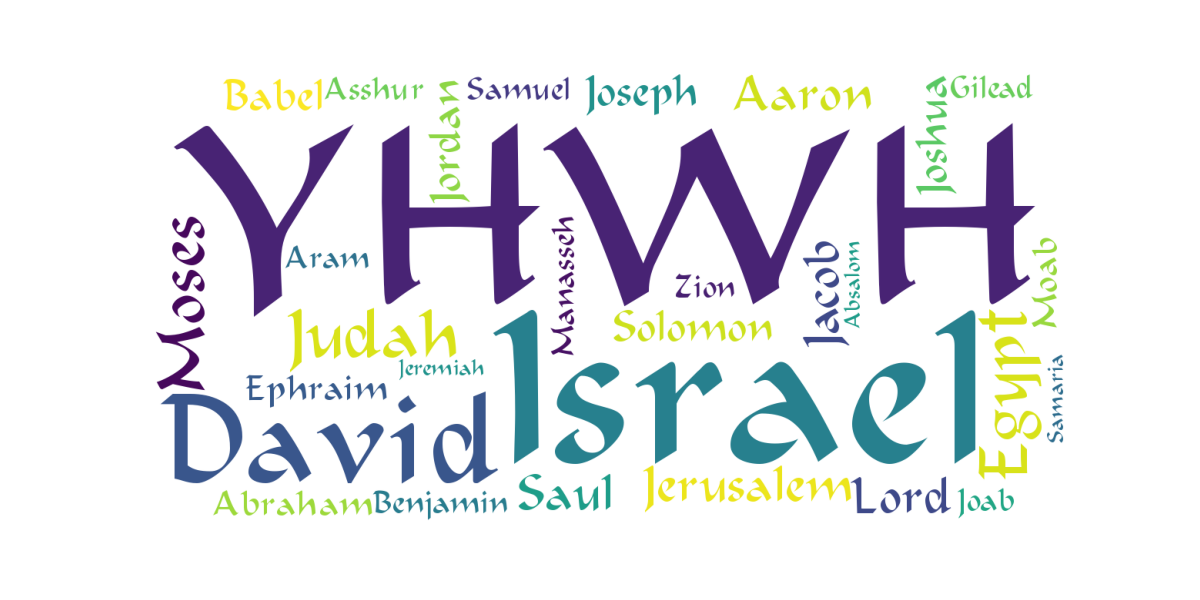
To me this is very exciting, with just some lines of code (and of course standing in the shoulders of giants that made TF possible and the BHSA dataset), we are able to perform simple tasks like this.
Most frequent verbs #
I want more, so lets repeat the same procedure but with verbs now. And lets increase the amount of verbs to 100 verbs sorted by frequency.
df_verbs = get_lexeme_by_type('verb')
df_cloud = df_verbs.sort_values(by=['freq_lex'], ascending=False)
print_df = export_df_to_org_table(df_cloud, 100)
w | freq_lex | lex_utf8 | gloss |
---|---|---|---|
1437621 | 5307 | אמר | say |
1437611 | 3561 | היה | be |
1437637 | 2629 | עשה | make |
1437773 | 2570 | בוא | come |
1437669 | 2010 | נתן | give |
1437760 | 1547 | הלך | walk |
1437623 | 1298 | ראה | see |
1437812 | 1159 | שמע | hear |
1438048 | 1138 | דבר | speak |
1437900 | 1082 | ישב | sit |
1437657 | 1068 | יצא | go out |
1437844 | 1038 | שוב | return |
1437764 | 965 | לקח | take |
1437799 | 944 | ידע | know |
1437724 | 890 | עלה | ascend |
1437851 | 847 | שלח | send |
1437769 | 835 | מות | die |
1437768 | 810 | אכל | eat |
1437628 | 732 | קרא | call |
1437894 | 653 | נשא | lift |
1437884 | 629 | קום | arise |
1437736 | 583 | שים | put |
1438023 | 548 | עבר | pass |
1438442 | 521 | עמד | stand |
1437899 | 500 | נכה | strike |
1437767 | 494 | צוה | command |
1437835 | 492 | ילד | bear |
1437766 | 468 | שמר | keep |
1437775 | 453 | מצא | find |
1437776 | 434 | נפל | fall |
1438193 | 377 | ירד | descend |
1437782 | 376 | בנה | build |
1437819 | 370 | נגד | report |
1439047 | 347 | מלך | be king |
1437816 | 332 | ירא | fear |
1437682 | 327 | ברך | bless |
1438467 | 316 | ענה | answer |
1438536 | 303 | פקד | miss |
1438046 | 297 | סור | turn aside |
1437685 | 292 | מלא | be full |
1438495 | 290 | חזק | be strong |
1437722 | 288 | עבד | work, serve |
1438067 | 288 | כרת | cut |
1437853 | 283 | חיה | be alive |
1438581 | 283 | איב | be hostile |
1438231 | 281 | קרב | approach |
1438522 | 237 | חטא | miss |
1438022 | 231 | זכר | remember |
1438347 | 231 | ירש | trample down |
1438906 | 225 | בקש | seek |
1437684 | 224 | רבה | be many |
1439718 | 223 | כתב | write |
1438074 | 217 | שתה | drink |
1439180 | 216 | כון | be firm |
1437788 | 214 | עזב | leave |
1438225 | 214 | נטה | extend |
1438878 | 213 | נצל | deliver |
1438479 | 212 | שכב | lie down |
1437866 | 212 | יסף | add |
1438565 | 211 | אהב | love |
1437706 | 206 | כלה | be complete |
1439443 | 205 | ישע | help |
1438002 | 204 | אסף | gather |
1438392 | 202 | שפט | judge |
1438251 | 193 | יכל | be able |
1438014 | 188 | רום | be high |
1438077 | 188 | גלה | uncover |
1439563 | 186 | אבד | perish |
1438554 | 185 | שבע | swear |
1438643 | 171 | שאל | ask |
1437711 | 171 | קדש | be holy |
1439420 | 171 | לחם | fight |
1438427 | 170 | חוה | bow down |
1439181 | 170 | בין | understand |
1437962 | 169 | בחר | examine |
1437869 | 168 | רעה | pasture |
1437885 | 167 | הרג | kill |
1438064 | 164 | דרש | inquire |
1438964 | 162 | טמא | be unclean |
1437750 | 161 | סבב | turn |
1438306 | 159 | נוס | flee |
1439475 | 154 | שמח | rejoice |
1438016 | 152 | כסה | cover |
1437797 | 150 | נגע | touch |
1438486 | 147 | שבר | break |
1438182 | 146 | נסע | pull out |
1438240 | 146 | הלל | praise |
1438652 | 146 | שנא | hate |
1437981 | 145 | שחת | destroy |
1438318 | 143 | רדף | pursue |
1438727 | 143 | חנה | encamp |
1437765 | 141 | נוח | settle |
1438323 | 140 | קרא | encounter |
1438461 | 135 | פנה | turn |
1438010 | 135 | פתח | open |
1437937 | 134 | חלל | defile |
1438923 | 134 | זבח | slaughter |
1438020 | 133 | שאר | remain |
1438373 | 133 | קבר | bury |
1437856 | 129 | שכן | dwell |
Here we can see the id of the lexeme (w), the frequency (freq_lex), the utf8 representation of the word without vowel pointing and the english meaning used for the translation. All automatically, pretty cool isn’t it?
Now lets create word clouds out of those verbs.
First, the top 30 verbs in Hebrew:
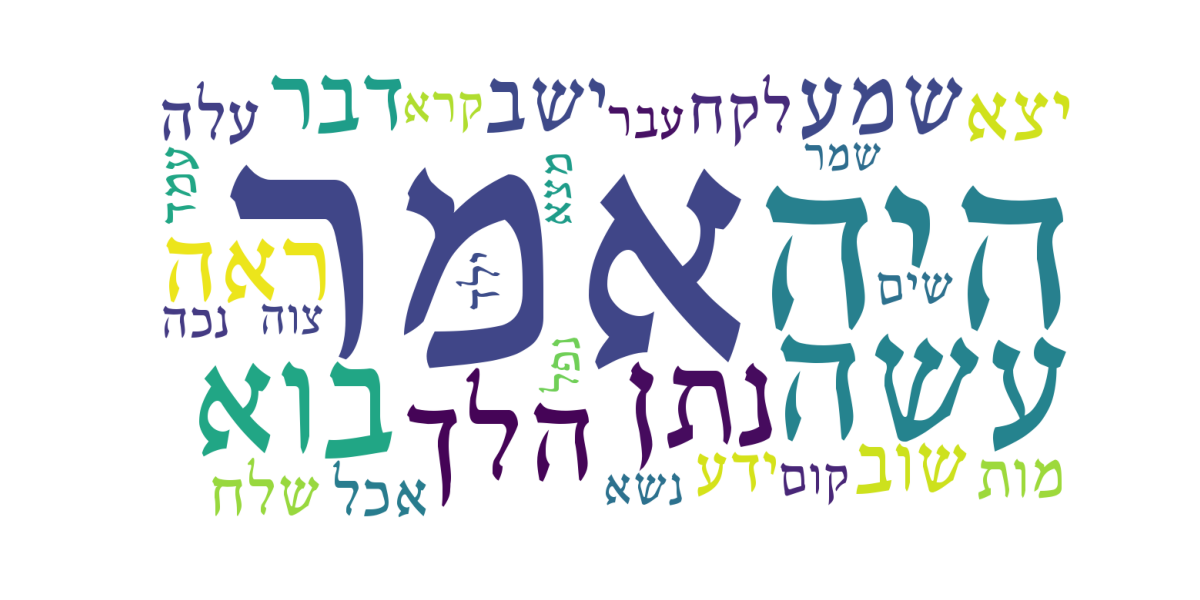
Now lets try the 50 most common verbs in Hebrew:
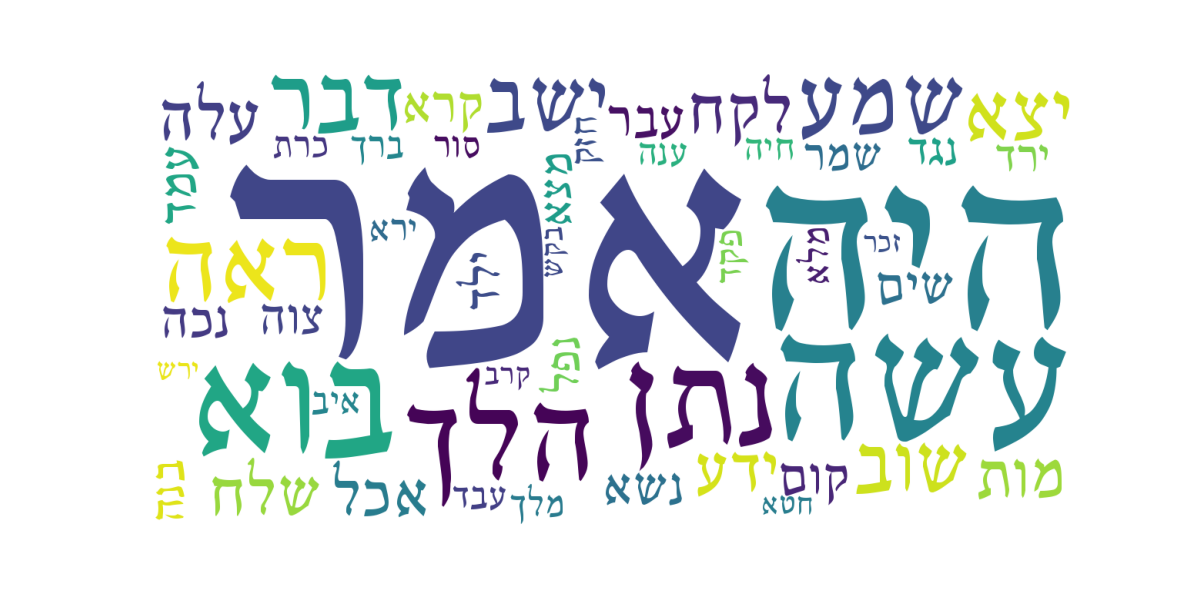
And now lets increase the amount to 100 verbs in Hebrew:
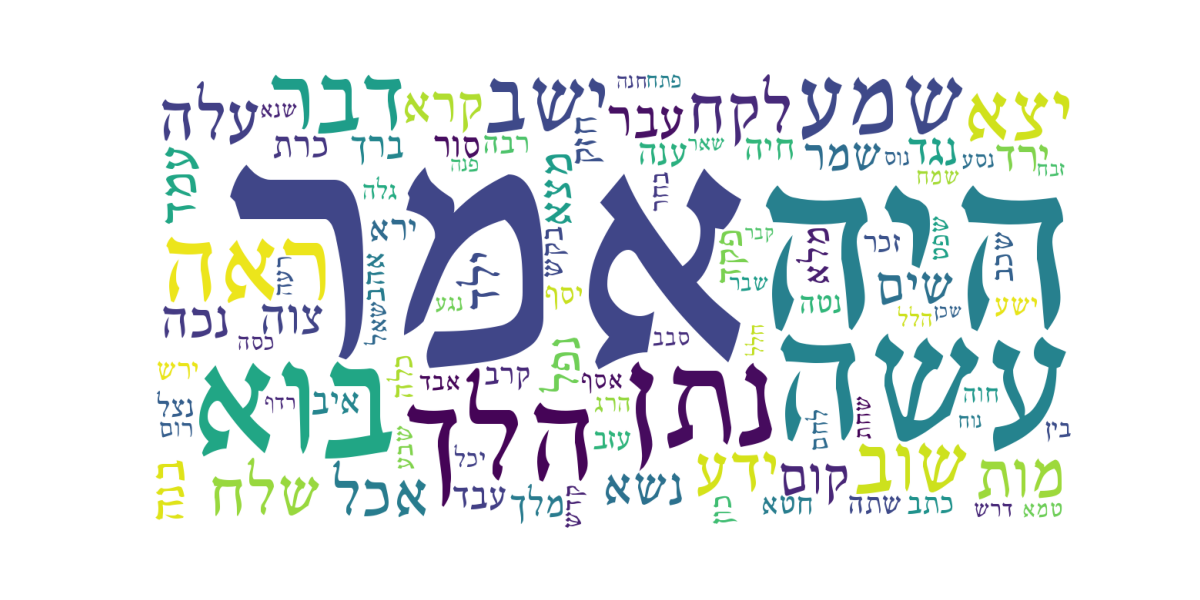
Now lets generate a cloud words image for the top 30 verbs in English:
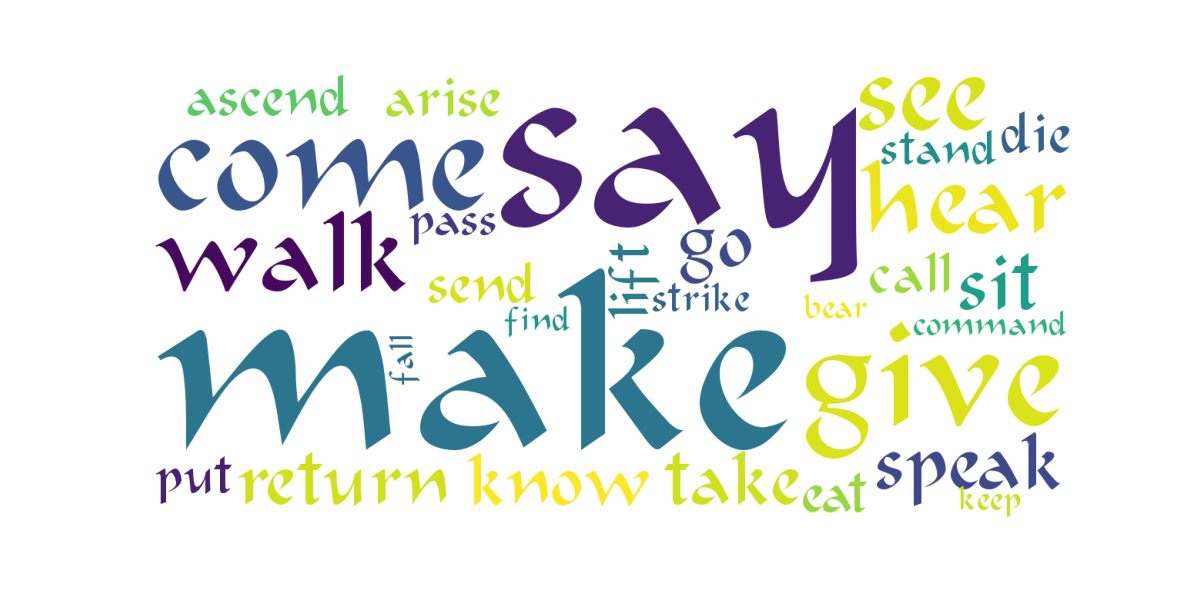
Similarly, for the 50 top verbs in English:
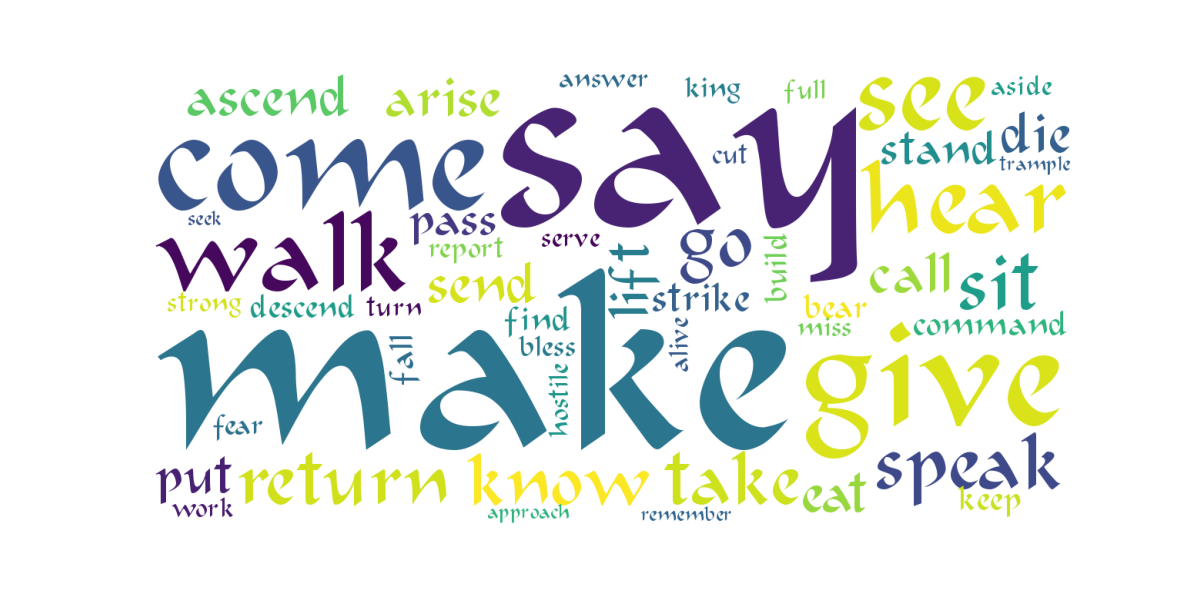
And finally, the top 100 top verbs in English:
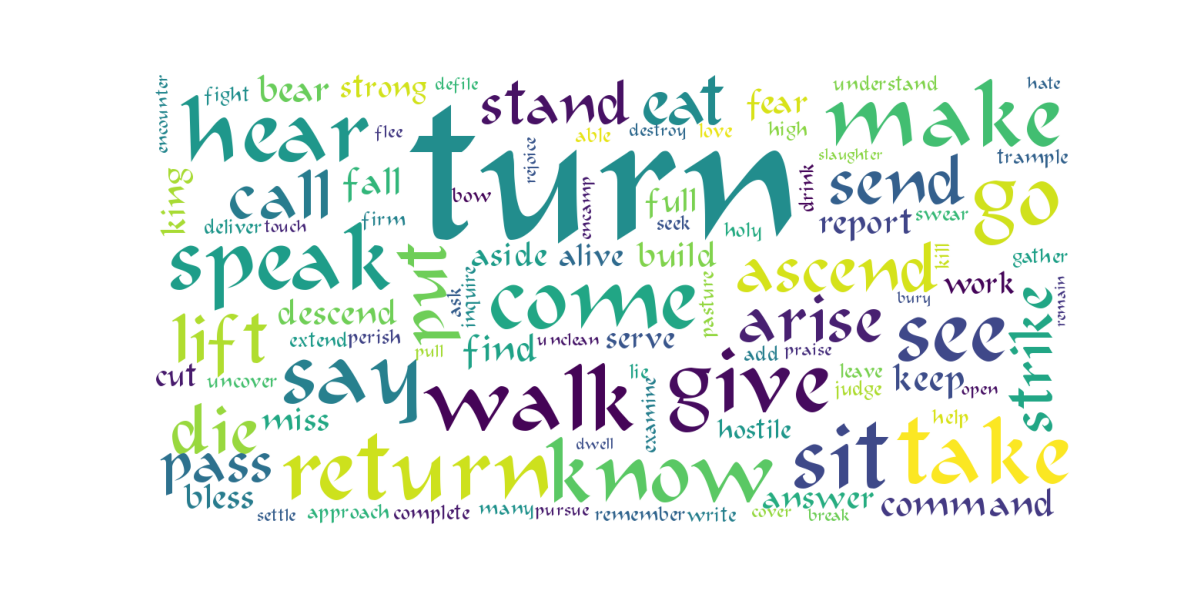
I have noticed something “unexpected” here with vowel pointing1. The word
“Turn” is the bigger one in english, yet is not the most frequent word in the
table. Why is that? Although I am not completely sure about it, I believe it is
due that it appears twice on the list since there are two Hebrew words
translated as turn with different amount of frequencies. I have not taken that
into account in the code. This doesn’t happen with the Hebrew words since the
frequency would already be increased in the freq_lex
column.
To solve this, I would probably have to parse the pandas dataframe to manually sum the frequencies of the same english word. But I won’t do that right now since I am more curious about exploring other words.
Furthermore, the “mistake” is rather interesting and I believe it speaks volumes in itself, since I truly believe that the will of the heart of our Father goes towards teshuva indeed, turn of our own ways. Seeing this unexpected event was rather interesting to me.
Most frequent substantives #
df = get_lexeme_by_type('subs')
df_cloud = df.sort_values(by=['freq_lex'], ascending=False)
print_df = export_df_to_org_table(df_cloud, 100)
w | freq_lex | lex_utf8 | gloss |
---|---|---|---|
1437679 | 5412 | כל | whole |
1437836 | 4937 | בן | son |
1437605 | 2601 | אלהים | god(s) |
1438272 | 2523 | מלך | king |
1437610 | 2504 | ארץ | earth |
1437630 | 2304 | יום | day |
1437787 | 2186 | איש | man |
1437616 | 2127 | פנה | face |
1437987 | 2063 | בית | house |
1438194 | 1866 | עם | people |
1437852 | 1618 | יד | hand |
1438181 | 1441 | דבר | word |
1437789 | 1217 | אב | father |
1437903 | 1090 | עיר | town |
1437634 | 970 | אחד | one |
1437801 | 887 | עין | eye |
1437662 | 876 | שנה | year |
1437747 | 864 | שם | name |
1438084 | 800 | עבד | servant |
1437721 | 788 | אין | <NEG> |
1437783 | 781 | אשה | woman |
1437664 | 768 | שנים | two |
1437674 | 754 | נפש | soul |
1438327 | 750 | כהן | priest |
1437942 | 715 | אחר | after |
1437861 | 706 | דרך | way |
1437867 | 629 | אח | brother |
1437940 | 602 | שלש | three |
1437973 | 601 | לב | heart |
1437746 | 599 | ראש | head |
1437944 | 588 | בת | daughter |
1437620 | 582 | מים | water |
1437941 | 579 | מאה | hundred |
1438017 | 558 | הר | mountain |
1438101 | 555 | גוי | people |
1437691 | 553 | אדם | human, mankind |
1437946 | 506 | חמש | five |
1437640 | 505 | תחת | under part |
1437813 | 505 | קול | sound |
1437889 | 498 | פה | mouth |
1438530 | 492 | אלף | thousand |
1437897 | 490 | שבע | seven |
1437932 | 490 | עוד | duration |
1437707 | 486 | צבא | service |
1439456 | 469 | קדש | holiness |
1437745 | 454 | ארבע | four |
1437854 | 438 | עולם | eternity |
1438456 | 422 | משפט | justice |
1437608 | 421 | שמים | heavens |
1438238 | 421 | שר | chief |
1437636 | 418 | תוך | midst |
1437859 | 412 | חרב | dagger |
1437627 | 407 | בין | interval |
1438247 | 403 | כסף | silver |
1437645 | 401 | מקום | place |
1438050 | 401 | מזבח | altar |
1437648 | 396 | ים | sea |
1437752 | 389 | זהב | gold |
1437618 | 378 | רוח | wind |
1438381 | 378 | אש | fire |
1438578 | 376 | נאם | speech |
1438471 | 374 | שער | gate |
1437886 | 360 | דם | blood |
1437913 | 345 | אהל | tent |
1438610 | 336 | סביב | surrounding |
1438449 | 335 | אדון | lord |
1437654 | 330 | עץ | tree |
1438645 | 325 | כלי | tool |
1437716 | 320 | שדה | open field |
1437965 | 315 | עשרים | twenty |
1438523 | 315 | נביא | prophet |
1437970 | 313 | רעה | evil |
1438278 | 308 | מלחמה | war |
1437704 | 300 | מאד | might |
1437842 | 298 | לחם | bread |
1438040 | 297 | עת | time |
1437882 | 293 | חטאת | sin |
1438051 | 286 | עלה | burnt-offering |
1438001 | 284 | ברית | covenant |
1438005 | 284 | חדש | month |
1437729 | 277 | אף | nose |
1438239 | 274 | פרעה | pharaoh |
1437951 | 274 | שש | six |
1437870 | 274 | צאן | cattle |
1437755 | 273 | אבן | stone |
1438299 | 270 | מדבר | desert |
1437781 | 270 | בשר | flesh |
1439127 | 252 | מטה | staff |
1438519 | 252 | לבב | heart |
1437990 | 247 | אמה | cubit |
1438038 | 247 | רגל | foot |
1438498 | 245 | חסד | loyalty |
1438977 | 244 | חיל | power |
1438338 | 240 | נער | boy |
1438145 | 240 | גבול | boundary |
1438372 | 237 | שלום | peace |
1438328 | 235 | אל | god |
1437956 | 235 | מעשה | deed |
1437893 | 231 | עון | sin |
1437653 | 229 | זרע | seed |
Most frequent conjunctions #
df = get_lexeme_by_type('conj')
df_cloud = df.sort_values(by=['freq_lex'], ascending=False)
print_df = export_df_to_org_table(df_cloud, 100)
w | freq_lex | lex_utf8 | gloss |
---|---|---|---|
1437609 | 50272 | ו | and |
1437638 | 5500 | אשר | <relative> |
1437624 | 4483 | כי | that |
1437878 | 1068 | אם | if |
1443538 | 731 | ו | and |
1438644 | 320 | או | or |
1437964 | 138 | ש | <relative> |
1437798 | 133 | פן | lest |
1438420 | 22 | לו | if only |
1445238 | 16 | הן | if |
1439657 | 15 | זו | <relative> |
1438910 | 14 | לולא | unless |
1445279 | 7 | להן | but |
1445073 | 2 | אלו | if |
1441905 | 1 | אין | if |
Most frequent adjectives #
df = get_lexeme_by_type('adjv')
df_cloud = df.sort_values(by=['freq_lex'], ascending=False)
print_df = export_df_to_org_table(df_cloud, 100)
w | freq_lex | lex_utf8 | gloss |
---|---|---|---|
1437665 | 526 | גדול | great |
1437703 | 469 | טוב | good |
1437969 | 424 | רב | much |
1437742 | 347 | רע | evil |
1439474 | 292 | לוי | Levite |
1438130 | 288 | פלשתי | Philistine |
1438464 | 262 | רשע | guilty |
1437675 | 239 | חי | alive |
1437978 | 206 | צדיק | just |
1438044 | 182 | ראשון | first |
1438444 | 181 | זקן | old |
1437934 | 166 | אחר | other |
1437642 | 156 | שני | second |
1439171 | 138 | חכם | wise |
1439676 | 119 | ישר | right |
1439730 | 116 | קדוש | holy |
1437708 | 98 | שביעי | seventh |
1438003 | 96 | טהר | pure |
1438975 | 94 | חלל | pierced |
1437979 | 91 | תמים | complete |
1440077 | 88 | טמא | unclean |
1438135 | 87 | אמרי | Amorite |
1438568 | 85 | רחוק | remote |
1437658 | 83 | שלישי | third |
1442586 | 82 | יהודי | Jewish |
1437697 | 82 | זכר | male |
1438499 | 76 | קרוב | near |
1439788 | 75 | עני | humble |
1438144 | 73 | כנעני | Canaanite |
1444427 | 70 | כסיל | insolent |
1439969 | 70 | זר | strange |
1442699 | 64 | כשדי | Chaldean |
1439798 | 61 | אביון | poor |
1439463 | 56 | חזק | strong |
1437671 | 56 | רביעי | fourth |
1437667 | 54 | קטן | small |
1438329 | 53 | עליון | upper |
1439418 | 52 | חדש | new |
1438949 | 51 | אחרון | at the back |
1438574 | 49 | ירא | afraid |
1439174 | 48 | דל | poor |
1438386 | 48 | חתי | Hittite |
1438083 | 47 | קטן | small |
1438883 | 45 | נכרי | foreign |
1438233 | 43 | יפה | beautiful |
1438641 | 43 | נקי | innocent |
1438230 | 41 | כבד | heavy |
1438134 | 41 | יבוסי | Jebusite |
1438018 | 37 | גבה | high |
1438770 | 37 | מר | bitter |
1439209 | 36 | קשה | hard |
1441758 | 35 | יקר | rare |
1438416 | 35 | ערל | uncircumcised |
1438309 | 34 | עברי | Hebrew |
1437686 | 34 | חמישי | fifth |
1439960 | 33 | ימני | right-hand |
1441098 | 32 | חסיד | loyal |
1442307 | 32 | פנימי | inner |
1440562 | 31 | צר | narrow |
1438455 | 31 | עצום | mighty |
1439795 | 31 | שמיני | eighth |
1438235 | 30 | מצרי | Egyptian |
1438855 | 29 | לבן | white |
1438030 | 29 | עשירי | tenth |
1438376 | 28 | שלם | complete |
1437705 | 28 | ששי | sixth |
1439654 | 27 | אדיר | mighty |
1440033 | 27 | נדיב | willing |
1439212 | 26 | כן | correct |
1443017 | 26 | אויל | foolish |
1439472 | 26 | עור | blind |
1442311 | 25 | חיצון | external |
1440433 | 25 | כשי | Ethiopian |
1440474 | 25 | בצור | fortified |
1438137 | 25 | חוי | Hivite |
1438253 | 23 | פרזי | Perizzite |
1439979 | 23 | עשיר | rich |
1438509 | 22 | צעיר | little |
1439345 | 22 | עז | strong |
1438606 | 21 | מלא | full |
1440818 | 21 | עמוני | Ammonite |
1440434 | 21 | ענו | humble |
1440919 | 21 | שמח | joyful |
1438972 | 21 | רחב | wide |
1442916 | 20 | עריץ | ruthless |
1441742 | 20 | רעב | hungry |
1438266 | 19 | חטא | sinful |
1445272 | 19 | רב | great |
1440889 | 19 | רענן | luxuriant |
1437997 | 19 | תחתי | lower |
1440295 | 18 | תשיעי | ninth |
1439610 | 18 | שכיר | hired |
1441042 | 18 | נבל | stupid |
1440613 | 18 | ראובני | Reubenite |
1440162 | 17 | עמק | deep |
1439751 | 17 | חפשי | free |
1438706 | 17 | עיף | faint |
1440167 | 17 | שפל | low |
1441912 | 16 | חסר | lacking |
1441561 | 16 | אביר | strong |
Most frequent pronouns #
df = get_lexeme_by_type('prps')
df_cloud = df.sort_values(by=['freq_lex'], ascending=False)
print_df = export_df_to_org_table(df_cloud, 100)
w | freq_lex | lex_utf8 | gloss |
---|---|---|---|
1437749 | 1394 | הוא | he |
1437998 | 874 | אני | i |
1437820 | 747 | אתה | you |
1437753 | 485 | היא | she |
1437817 | 359 | אנכי | i |
1437967 | 291 | המה | they |
1438066 | 283 | אתם | you |
1437807 | 269 | הם | they |
1438256 | 120 | אנחנו | we |
1438234 | 57 | את | you |
1437961 | 48 | הנה | they |
1445253 | 16 | אנה | I |
1445354 | 15 | אנתה | you |
1445320 | 15 | הוא | he |
1445913 | 9 | המו | they |
1445260 | 7 | היא | she |
1439211 | 5 | נחנו | we |
1438874 | 4 | אתנה | you |
1445496 | 4 | אנחנא | we |
1445384 | 3 | המון | they |
1445421 | 3 | אנון | they |
1445255 | 1 | אנתון | you |
1443994 | 1 | אתן | you |
1445760 | 1 | אנין | they |
Most frequent adverbs #
df = get_lexeme_by_type('advb')
df_cloud = df.sort_values(by=['freq_lex'], ascending=False)
print_df = export_df_to_org_table(df_cloud, 100)
w | freq_lex | lex_utf8 | gloss |
---|---|---|---|
1437737 | 834 | שם | there |
1437804 | 769 | גם | even |
1438351 | 577 | כה | thus |
1437641 | 546 | כן | thus |
1437850 | 433 | עתה | now |
1437896 | 200 | לכן | therefore |
1438021 | 161 | אך | only |
1437936 | 141 | אז | then |
1437796 | 133 | אף | even |
1437974 | 109 | רק | only |
1438250 | 96 | יחדו | together |
1438489 | 82 | פה | here |
1445293 | 57 | אדין | then |
1438375 | 50 | הנה | here |
1439618 | 50 | יומם | by day |
1438389 | 45 | אולי | perhaps |
1439587 | 37 | ככה | thus |
1438807 | 32 | חנם | in vain |
1440400 | 25 | פתאם | suddenly |
1438796 | 19 | אולם | but |
1438485 | 16 | הלאה | further |
1438912 | 16 | ריקם | with empty hands |
1440112 | 13 | פנימה | within |
1445334 | 13 | כען | now |
1438403 | 12 | הלם | hither |
1442642 | 9 | אמנם | really |
1445336 | 8 | כן | thus |
1445965 | 7 | אספרנא | exactly |
1438081 | 7 | אחרנית | backwards |
1445901 | 5 | כנמא | thus |
1438451 | 5 | אמנם | really |
1445348 | 5 | ברם | but |
1445714 | 4 | אף | also |
1445973 | 4 | תמה | there |
1445250 | 3 | להן | therefore |
1445917 | 3 | כענת | now |
1443334 | 3 | דומם | silently |
1444590 | 3 | אזי | then |
1445237 | 2 | אזדא | publicly known |
1444976 | 2 | להן | therefore |
1438528 | 2 | אמנה | indeed |
1445939 | 1 | כעת | now |
1445703 | 1 | טות | fastingly |
1445771 | 1 | כה | here |
1442542 | 1 | מסח | alternatively |
1445670 | 1 | עלא | above |
1445537 | 1 | אחרין | at last |
1445492 | 1 | צדא | really |
1445251 | 1 | תנינות | again |
1445069 | 1 | עדן | hitherto |
1445068 | 1 | עדנה | hitherto |
1444323 | 1 | קדרנית | mourningly |
1446033 | 1 | אדרזדא | with zeal |
Most frequent demonstrative pronouns #
df = get_lexeme_by_type('prde')
df_cloud = df.sort_values(by=['freq_lex'], ascending=False)
print_df = export_df_to_org_table(df_cloud, 100)
w | freq_lex | lex_utf8 | gloss |
---|---|---|---|
1437938 | 1177 | זה | this |
1437712 | 746 | אלה | these |
1437784 | 604 | זאת | this |
1443532 | 58 | דנה | this |
1445488 | 14 | אלך | these |
1441693 | 11 | זה | this |
1438482 | 9 | אל | these |
1445926 | 7 | דך | that |
1441583 | 7 | לז | this there |
1445595 | 6 | דא | this |
1445971 | 6 | דך | that |
1445409 | 5 | אלין | these |
1445363 | 3 | דכן | that |
1444075 | 2 | זו | this |
1438655 | 2 | לזה | this there |
1443546 | 1 | אלה | these |
1445970 | 1 | אל | these |
1443998 | 1 | לזו | this there |
Most frequent interrogative particles #
df = get_lexeme_by_type('inrg')
df_cloud = df.sort_values(by=['freq_lex'], ascending=False)
print_df = export_df_to_org_table(df_cloud, 100)
w | freq_lex | lex_utf8 | gloss |
---|---|---|---|
1437821 | 743 | ה | <interrogative> |
1437877 | 178 | למה | why |
1438738 | 72 | מדוע | why |
1438719 | 61 | איך | how |
1438443 | 45 | איה | where |
1438846 | 43 | מתי | when |
1438396 | 41 | אן | whither |
1437815 | 39 | אי | where |
1440801 | 17 | איכה | how |
1438803 | 17 | אין | whence |
1439081 | 10 | איפה | where |
1445345 | 6 | ה | <interrogative> |
1445029 | 4 | איככה | how |
1444096 | 3 | אהי | where |
1445070 | 2 | אי | how |
1445785 | 2 | היך | how |
1441950 | 1 | אל | where |
1442508 | 1 | איכה | how |
- I will have to double check this. What I understood from the documentation of the API is that the lexemes do not have vowel pointing, however the shin/sin seems to have diacritics so I had to remove the diacritics of each word, because the word cloud was not properly rendered with diacritics.